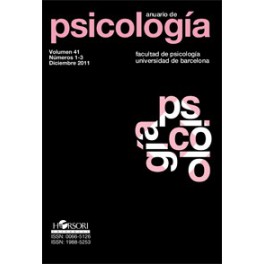
- Sacar de mis favoritos
- Agregar este producto a mis favoritos
- Imprimir
AP 41- Retaining principal components for discrete variables
Antonio Solanas, Rumen Manolov, David Leiva, University of Barcelona, María Marta Richard’s, National University of Mar de Plata, Argentina
ISBN: 1988-5253 ·
2011 · 16.5x23.5 cm
Siganos